Data Science Vs Data Analysis - How data-driven decision-making is important for D2C Success?
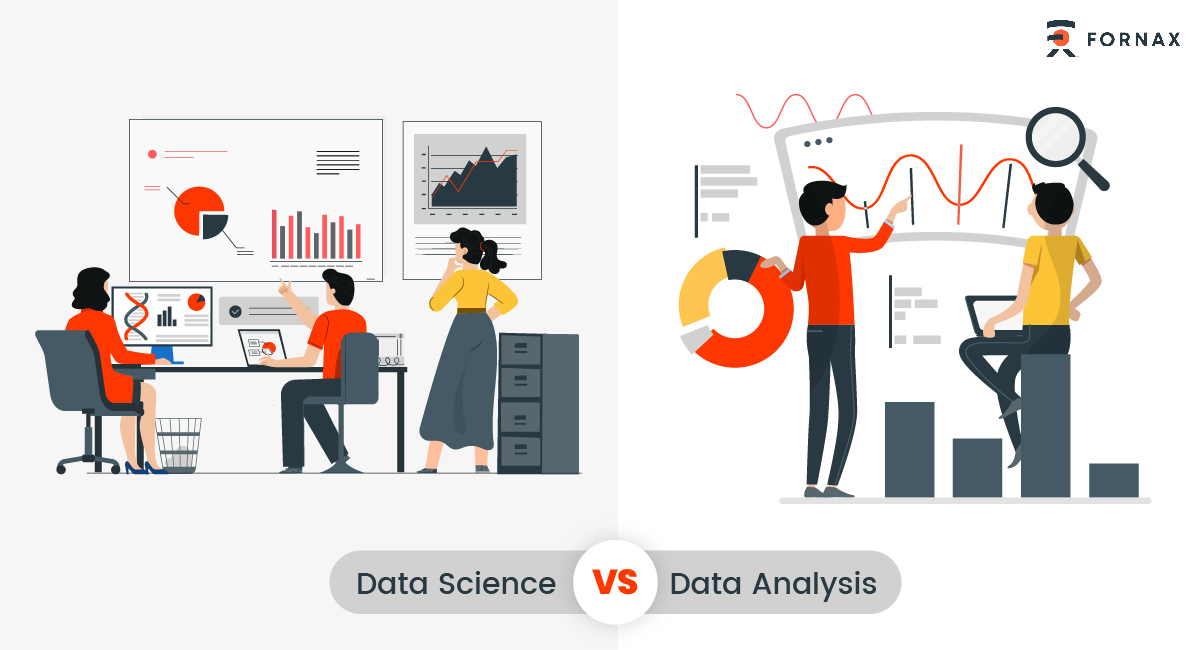
Introduction
The most significant distinction between conducting business in the 20th and 21st centuries can probably be summed up in one word: data.
Data’s increasing prominence over the past ten years has changed our world in ways we are only now beginning to fully understand. Data are now seen as a valuable resource in most companies and are seen as the key to gaining a competitive edge and implementing disruptive tactics.
Data science and analytics have traditionally been the domain of specialised academics. But now that big data is flourishing, automation and analytics software are on the rise, everyone can access data, and even the smallest businesses can use it. However, this means that an increasing number of business professionals now require the necessary knowledge and abilities to opt for data effectively.
Although working with and modifying data is a part of both the data science and data analytics professions, they are not equivalent.
What is data science?
Data Science is the process of cleansing, preparing, and aligning the data in a manner comparable to knowledge discovery in databases. It combines mathematics, machine learning, statistics, problem-solving, programming, and the capacity to look at things differently (KDD).
The main goal is to assist businesses in transforming their large amounts of big data into insightful information that can be used. Data science is not domain-specific, although its key drivers are computer science and machine learning.
What is data analysis?
The term "data analysis" describes the method to find answers, draw conclusions, and spot trends. Depending on the sort of analysis being done, different tools, methodologies, and frameworks are used to accomplish this. Extracting usable information from data and making decisions based on that analysis are the goals of data analysis.
To give a simple example of data analysis in everyday life, every time we make a decision, we consider the past and future to make a particular choice. This is nothing more than evaluating what happened or what might happen and making choices in light of it. Today, data analysis refers to the same process an analyst uses for commercial goals.
Data Science Vs Data Analysis
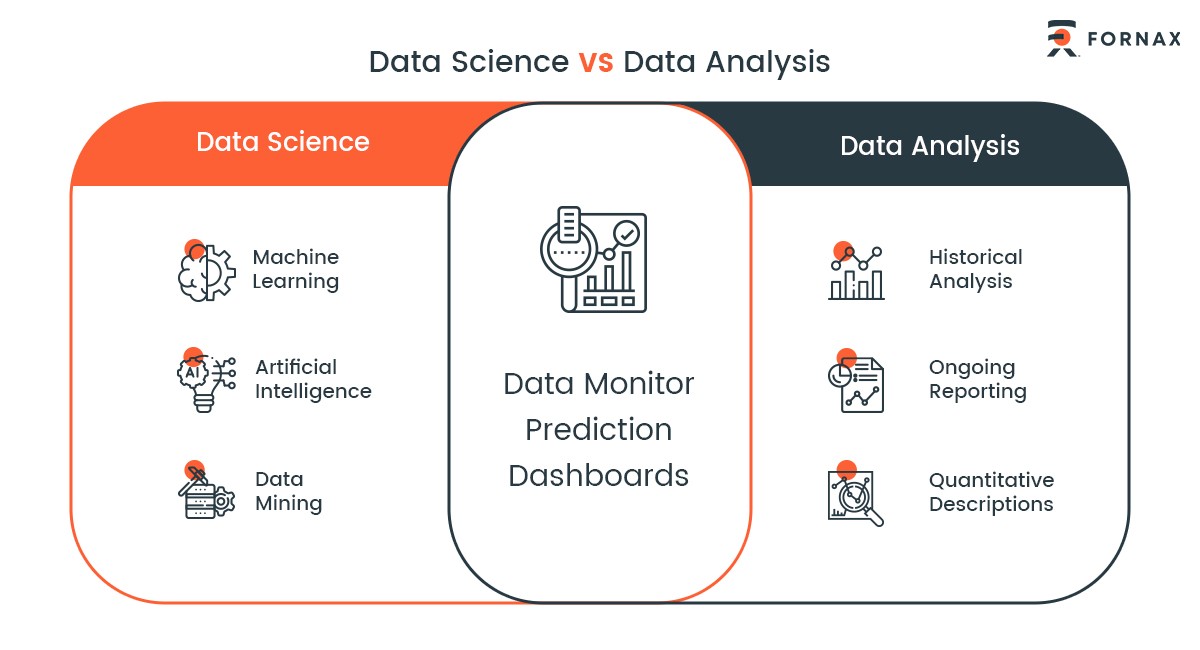
As mentioned above, the primary distinction between data science and data analysis is the end goal: when data analysis frequently concentrates on a narrow area (such as sales or e-commerce), data scientists adopt a systemic perspective on how an organisation functions.
Many people who work in the data industry first train as data analysts before transitioning to data science. Even if the distinction between the two can be indefinite at times, we can broadly classify them as follows:
- Objectives
Data science and data analysis are distinguished by their fundamental objectives. Analysis has a propensity to look over and mine business-related data. Data science is designed to find relevant business problems and answers. The analysis's objective is to offer solutions that will enable corporations to decide more rapidly and wisely. It seeks out practical insights using readily available data. Data science, on the other hand, is largely concerned with identifying new questions that may not have been known before and then coming up with answers. Data science seeks to establish links that will assist define current issues and offer solutions for all times.
- Usage and techniques
Data science is associated with predictive modelling and machine learning, whereas use analytics is focused on contextualising past data. Algorithms and inference are both included in this multidisciplinary practice. However, certain more generic subfields of analysis and statistics are included in data analytics.
- Roles performed by Experts
Data analysts compile the information that their companies have collected from various sources. They conduct probing on the data to improve its communication. The task of data scientists is to unearth facts buried in the complex web of unstructured data.
- Career Prospects
These two occupations are currently among the most popular in business, notwithstanding the differences between them. Data scientists and analysts have comparable job paths. The latter should have an information science, or computer science background. The former should ideally enrol in undergraduate IT, mathematics, statistics, and computer science courses.
Role of data in improvising customer service
Customer experience is currently evolving into a highly competitive area of any business domain. There has been a considerable surge in client expectations, and data is growing tremendously. The complexity of the customer experience has increased across many sub-domains, including technical support, online help, campaigns, surveys, account management, billing, and payment.
Here is how data can improve customer service:
- Predicting desired products - Businesses can perform a predictive study of their consumers' interests and present them with products they are likely to buy with the aid of big data and machine learning technologies.
- AI-based hassle-free customer service - Automating and streamlining customer service is the most important and useful use of AI. An artificial intelligence (AI) application programme known as a virtual assistant carries out the user's instructions by understanding natural language voice commands.
- Making Smooth Virtual Experiences with the Help of Behavior Analytics - Big data analytics is used to examine customer purchasing habits and behaviour. While using a product or service, a consumer might be thinking about several things.
- Looking for AI-Enabled Customer Insights - Customer journey analytics enabled by AI can handle a lot of data. Complex customer analytics data that can be used to research customer behaviour can be handled by it.
How are data-driven decisions the key to D2C growth?
As D2C brand competition increases, mastering the art of personalisation is one method to put the business ahead of the competition. But how can you properly personalize in 2023?
Data and insights are straightforward solutions. You can increase engagement and keep customers satisfied in the short and long term by using data to drive personalisation.
To establish lasting relationships, D2C marketers must also produce content that is consistent with their target audience's values. Additionally, data-smart audience segmentation guarantees that marketing communications are tailored to the requirements and tastes of each target.
As soon as you begin to take data-driven, immediate action, continue to monitor and get feedback from your clients. Every new piece of information you learn allows you to keep improving the customer experience in this ongoing cycle.
The advantage of data-driven decision-making over other types of decision-making is that it guarantees that conclusions are grounded in reason and logic and are validated by numerical evidence. Decision-makers run the risk of being unintentionally influenced by variables like bias, external stimuli like other people's demands or opinions, or acting on assumptions, gut feelings, or theories if data is not used to guide judgements.
The use of data to affect decision-making has a number of advantages. Making decisions based on data is advantageous because:
- It is an objective process because it is based on figures and numerical data.
- Data-driven decision-making has the advantage of being easily examined in light of how certain decisions are implemented and how they affect different metrics.
- It makes more control possible. You have more influence over the direction of your business and the calibre of your decisions when you use data-driven decision-making.
- It makes you more agile. One can spot business prospects earlier, recognise problems more quickly and react to market developments more quickly.
Key Takeaways
Making decisions based on data isn't only a new trend that companies are trying to adopt in order to stay current. It is a procedure that has been scientifically proven and offers firms a methodical and objective approach to decision-making that can help to boost process efficiency, decision effectiveness, workplace productivity, and corporate growth. You may be confident that you're making the right choice if you're thinking about using data to your advantage to position your business for success.
Get in touch with our Fornax team today!